This book excerpt was written by either a human or by artificial intelligence. Try to guess which one:
A last view of the poison path is that it is a new way of thinking about a long-term future for the human species. This view is supported by the recent realization that humanity has already entered a period of mass extinction.
If the poison path can carve a route through the Western Umwelt, and reveal itself through a process of embedding that transforms the consciousness of the West, then the poison path may be the path to the long-term survival of the human species.
The answer? It was written by AI, with human prompts.
Pharmako-AI, published in August 2020, was the first book co-written with artificial intelligence. The project emerged from author Kenric Allado-McDowell’s personal diary entries used as prompts for GPT-3, one of the world’s most advanced language AIs.
{{guide-202403-2="/rt-components"}}
AI has continued to evolve and grow, despite debates about the ethical implications of its existence and the way it operates. With that growth, investment banks are starting to find their own efficiencies with AI and machine learning.
Since JPMorgan Chase announced their LOXM AI algorithm in 2017, investment banks have been gingerly increasing their own use of machine learning to execute trades, manage relationships, and deal with allocation requests.
But what does that actually look like, right now? “Artificial intelligence” and “machine learning” are such broadly used terms it can be difficult to separate actual applications from wishful thinking. Here we’ll catch you up on the status of AI within investment banking, so you can assess where you can use AI and ML to improve your workflows starting soon, not five years from now.
AI efficiencies: What investment banks stand to gain from adoption
AI technologies could “potentially unlock $1 trillion of incremental value for banks.” AI would unlock this value by speeding up human decision-making and increasing the likelihood that humans will make the right decisions that will lead to growth.
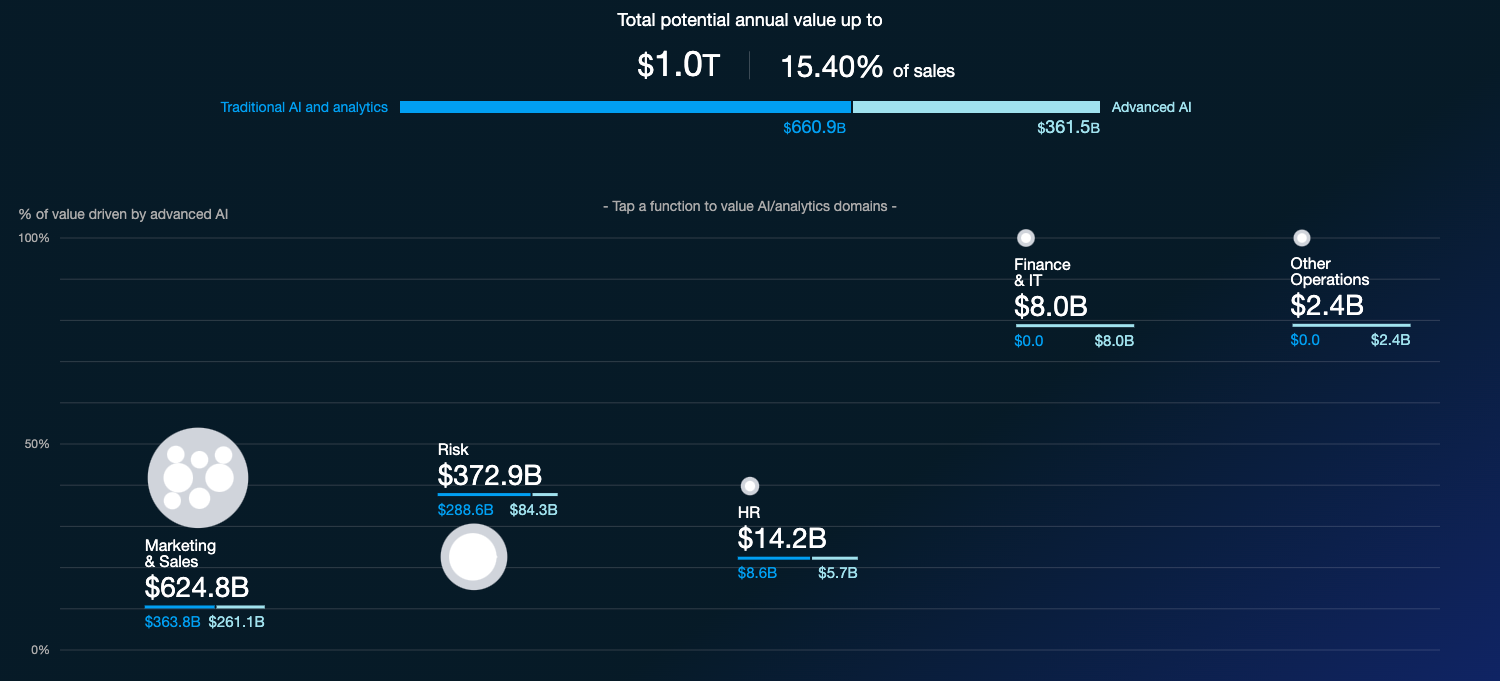
Source: McKinsey
While investment banks have been slow to adopt AI, the majority of financial services companies are now using it for risk management (56%) and revenue generation (52%).
More precisely, AI is improving decision-making in the following areas:
- Marketing and sales: Customer service, relationship management, promotion, budget allocation, personalized offerings, etc.
- Risk management: Modeling, fraud prevention
- Human resources: Productivity, hiring, and employee retention
Within each of these areas, investment banks can expect to see the following three main outcomes of AI-integrated decision-making:
- More revenue—by offering customers products or services more specific to their needs and building better customer and client relationships
- Lower overhead—by reducing human error and identifying optimal budget allocation across departments
- New opportunities—by analyzing market data in novel ways and managing the relationships needed to capitalize on partnerships
How AI is uncovering new opportunities in investment banking
More revenue and lower overhead are exciting outcomes to see on a balance sheet, but AI is also supporting front office teams by helping find new opportunities in investment banking.
The primary function of artificial intelligence is data analysis. The human brain can only analyze a small amount of data, and that’s part of what makes us so bad at predicting the future or keeping up with shifting consumer preferences. AI still has a long way to go before it can solve all of your pipeline problems, but it’s still making positive outcomes more likely.
Here are three main areas experts focus on when using AI to create new opportunities in investment banking:
Personalizing client service
Conversational AI, or chatbots, are one way to deliver personalized customer service in investment banking at scale. Chatbots use natural language processing to understand the needs of the customer and deliver the same kind of response a customer service agent would.
That said, chatbots aren’t yet widely used in banking—they currently make up only 13.5% of the AI vendor product offerings in the industry, and even the most advanced iterations are only handling basic requests. This is because natural language processing is still in its infancy. As it turns out, personalized service with a robot is really hard to execute!
Learn all about how you can analyze your data to personalize client experiences.
Still, some firms are making exciting advancements with internal investments in conversational AI. JPMorgan Chase, for example, developed their Contract Intelligence (COiN) chatbot to analyze legal documents. As compared to manual legal review of 12,000 commercial credit agreements in 360,000 hours, COiN reviewed the same amount of information in mere seconds without error.
While this technology hasn’t yet benefited external stakeholders, JPMorgan Chase is aggressively hiring audio AI specialists to scale their conversational AI research—so definitely keep an eye on them to lead the way in personalized service with AI.
Predicting investment risk and new markets
We mentioned before that JPMorgan Chase was leading the way in 2017 with the development of its LOXM algorithm, which conducted buying and selling activities across its equities business. The firm has since been publishing more research on a simulator it created “to assess the effect a market marker can have on equality of outcomes for consumer or retail traders.” Introducing machine learning to the risk assessment process empowers teams to make more accurate decisions, faster.
{{guide-202403-2="/rt-components"}}
This is because machine learning analyzes large swaths of data to monitor the market so it can eventually understand what causes stock prices to rise and fall. With enough of this data, artificial intelligence can more accurately predict outcomes humans may not have considered. With AI-driven risk assessment, firms will be able to:
- Reduce trader errors
- Trigger trades at the best possible price
- Recommend products based on personal risk tolerance
- Customize portfolios based on personal investment values
While no one investment bank seems to be doing this well with artificial intelligence at the moment, investments in AI fraud detection could pave the way for more exciting use cases in trading.
For example, Teradata helped Danske Bank improve their fraud detection process by removing 60% of their false positives per day, which allowed them to improve real fraud detection by 50%.
Fraud detection is the most widely used application of artificial intelligence in banking right now, and what investment banks learn from AI fraud detection will likely transfer to more accurate market predictions and risk assessment functions. But more research and development is needed before AI is used more broadly in those areas.
Making it easier to leverage relationships
One of the most exciting ways artificial intelligence can help investment banks uncover new opportunities is through relationship management. This is because the most powerful tool in any investment banker’s toolbox is their personal network.
If LinkedIn has taught us anything it’s that the more your network grows, the more unwieldy it can become. If it’s been years since an old colleague introduced you to that one person at the company you’re reaching out to, but you can’t remember their name (or anything else about them) off the top of your head, you can’t use that information to grow your business.
Relationship intelligence—a category of insights into your team’s network, business relationships, and client interactions that helps you find, manage, and close deals—combines with AI and ML to expand how bankers use technology.
Machine learning combined with relationship intelligence have created CRM platforms that can centralize, sort, and interpret contact recordas that have a ton of data associated with them. If your goal is to be as personable as possible with your communication, more data is good—but only when it’s searchable and in one place.
How investment bankers are using relationships CRMs to close more deals:
- Offloading data entry: Search contact databases and create contact records without time-consuming manual data entry—which causes a ton of inconsistency anyway.
- Showing clients depth of access to decision-makers: When clients approach your firm, they want to know that you have access to potential buyers. Relationship intelligence platforms score relationships so you can more quickly show clients a shortlist of contacts who you know you can reach.
- Automate follow-ups with built-in reminders: The more a network grows, the more difficult it can be to keep up consistent communication. By scouring email and calendar records, dealmakers can be sure to follow up with contacts in ways that keep relationships strong.
Humans are messy creatures, and decision-making and deals never run in straight lines. Artificial intelligence and machine learning technologies haven’t been able to eliminate human chaos. Still, the technologies are at least becoming skilled in cleaning up human messes when data is easy to classify.
For now, AI is making it possible to better organize your businesses and grow an expansive collective network you can rely on to close deals. Corporate Advisory Solutions cleaned up their own relationship database with Affinity’s relationship intelligence and used their more structured network data to expand their reach to new clients.
{{guide-202403-2="/rt-components"}}